Te Pei
(he/him)
Assistant Professor
Additional Departments/Affiliated Programs
Areas of Expertise/Research
- Geohazards and Georisks
- Geotechnical Engineering
- Machine Learning
Building
Steinman Hall
Office
103
Phone
(212) 650-5143
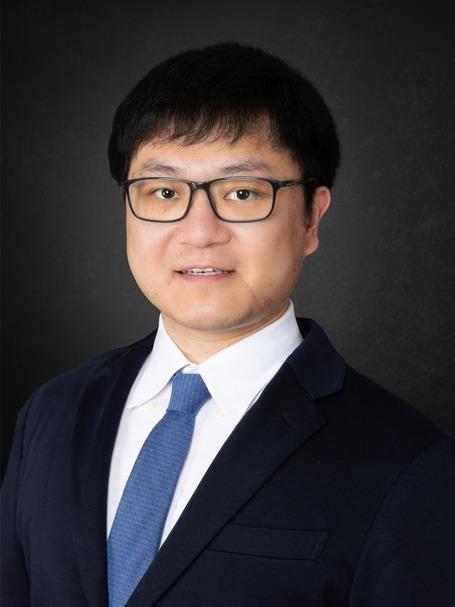
Te Pei
Education
PhD, Pennsylvania State University, 2023
BS, Oklahoma State University, 2017
BS, Southwest Jiaotong University, 2017
Courses Taught
Soil Mechanics (CE 34500)
Foundation Engineering (CE 59000)
Publications
Peer-Reviewed Journal Publications:
- Xiong, J., Pei, T., and Qiu, T. (2024). "A novel framework for spatial-temporal susceptibility prediction of rainfall-induced landslides: a case study in Western Pennsylvania." Remote Sensing. (Accepted)
- Pei, T., Qiu, T. (2024). “Landslide Susceptibility Mapping Using Physics-Guided Machine Learning: An Application in Colorado Front Range.” Acta Geotechnica.
- Pei, T., T. Qiu, and C. Shen. (2024). “Closure to ‘applying knowledge-guided machine learning to slope stability prediction.’” J. Geotech. Geoenviron. Eng., 150 (9).
- Li, Z., Pei, T., Ying, W., Zhang, R., Yoon, J., Dabo, I., Srubar, W., and Radlinska, A. (2024). “Can domain knowledge benefit machine learning for concrete property prediction?” Journal of the American Ceramic Society, 1-21.
- Pei, T., and Qiu, T. (2024). “Machine Learning with Monotonic Constraint for Geotechnical Engineering Applications: An Example of Slope Stability Prediction.” Acta Geotechnica.
- Pei, T., Qiu, T., and Shen, C. (2023). “Applying Physics-Guided Machine Learning to Slope Stability Prediction.” Journal of Geotechnical and Geoenvironmental Engineering, 149(10),04023089.
- Palese, M., Pei, T., Qiu, T., Zarembski, A. M., Shen, C., and Palese, J. W. (2023). “Hazard assessment framework for statistical analysis of cut slopes using track inspection videos and Geospatial Information.” Georisk: Assessment and Management of Risk for Engineered Systems and Geohazards, 17(4), 771–786.
- Pei, T., and Qiu, T. (2022). “A Numerical Investigation of Laterally Loaded Steel Fin Pile Foundation in Sand.” International Journal of Geomechanics, 22(7), 04022102.
- Pei, T., and Qiu, T. (2022). “DEM Investigation of Energy Dissipation at Particle Contacts in Granular Soil Under Cyclic Torsional Shear.” International Journal of Geomechanics, 22(4), 04022016.
- Nagendra, S., Kifer, D., Mirus, B., Pei, T., Lawson, K., Manjunatha, S., Li, W., Nguyen, H., Qiu, T., Tran, S. and Shen, C. (2022). “Constructing a Large-Scale Landslide Database Across Heterogeneous Environments Using Task-Specific Model Updates.” IEEE Journal of Selected Topics in Applied Earth Observations and Remote Sensing, 15, pp. 4349-4370.
- Pei, T., and Yang, X. (2018). “Compaction-Induced Stress in Geosynthetic-Reinforced Granular Base Course – A Discrete Element Model.” Journal of Rock Mechanics and Geotechnical Engineering, 10(4), 669–677.
Conference Publications/Presentations:
- Goldberg, R., Pei, T., Moshary, F., and Tian, Y. (2025). “Advancing Landslide Susceptibility Mapping Across Heterogeneous Regions with Deep Learning-Based Domain Adaptation.” in Proc. of Geotechnical Frontiers 2025 Conference. (Accepted)
- Pei, T., and Luo, N. (2025). “Prediction of Ultimate Bearing Capacity of Shallow Foundations Using Monotonically Constrained Neural Networks.” in Proc. of Geotechnical Frontiers 2025 Conference. (Accepted)
- Pei, T., Goldberg, R., Moshary, F., and Tian, Y. (2024). “Cross-Ecoregion Landslide Susceptibility Prediction Using Model-Agnostic Meta-Learning.” in Proc. AGU Fall Meeting Abstr., vol. 2024, 2024, Art. no. NH33C-2212.
- Pei, T., Maroufi, M., and Tian, Y. (2024). “A Physics-Informed Machine Learning Approach for Predicting Rainfall-Induced Landslides.” in Proc. AGU Fall Meeting Abstr., vol. 2024, 2024, Art. no. NH11C-2234.
- Xiong, J., Pei, T., and Qiu, T. (2024). “Spatiotemporal prediction of rainfall-induced landslides using machine learning techniques.” in Proc. of Geo-Shanghai International Conference 2024. (Best Paper Award)
- Li, Z., Pei, T., Ying, W., Zhang, R., Yoon, J., Dabo, I., Srubar, W., and Radlinska, A. (2024). “Simulation-assisted transfer learning for concrete strength prediction.” in Proc. of 77th RILEM Annual Week and the 1st Interdisciplinary Symposium on Smart & Sustainable Infrastructures (ISSSI 2023).
- Pei, T., Liu, J., Shen, C., and Kifer, D. (2023). “Impact of Cross-Validation Strategies on Machine Learning Models for Landslide Susceptibility Mapping: A Comparative Study.” in Proc. AGU Fall Meeting Abstr., vol. 2023, 2023, Art. no. NH13D-0717.
- Pei, T., and Qiu, T. (2023). “Landslide susceptibility mapping in Colorado Front Range, USA: a comparison of physics-based and data-driven approaches.” Accepted for publication in Proc. of 8th International Conference on Debris Flow Hazard Mitigation.
- Palese, M., Pei, T., Zarembskia, A., Qiu, T., Shen, C., and Palese, J. (2023). “Risk assessment framework for statistical analysis of cut slopes using track inspection videos and satellite imagery.” in Proc. of 2023 Georisk Conference.
- Pei, T., and Qiu, T. (2023). “Landslide susceptibility mapping using machine learning methods: a case study in Colorado Front Range, USA.” in Proc. of 2023 Geo-Congress Conference.
- Xiong, J., Pei, T., and Qiu, T. (2023). “A machine learning-based method with integrated physics knowledge for predicting bearing capacity of pile foundations.” in Proc. of 2023 Geo-Congress Conference.
- Pei, T., Nagendra, S., Banagere Manjunatha, S., He, G., Kifer, D., Qiu, T., and Shen, C. (2021). “Utilizing an interactive AI-empowered web portal for landslide labeling for establishing a landslide database in Washington state, USA.” in Proc. of EGU Gen. Assem. Conf. Abstr., 2021, Art. no. EGU21- 13974.
- Liu, J., Shen, C., Pei, T., Lawson, K., Kifer, D., Nagendra, S., and Manjunatha, B. (2021). “A new rainfall-induced deep learning strategy for landslide susceptibility prediction.” in Proc. of AGU Fall Meeting Abstr., vol. 2021, 2021, Art. no. NH35E-0504.
- Pei, T., Qiu. T., and Laman, J. (2020) “A numerical investigation of laterally loaded steel fin pile foundations.” in Proc. of 2020 Joint-Rail Conference, V001T08A012. New York: ASME.
- Pei, T., Nagendra, Manjunatha, B., S., He, G., Kifer, D., Qiu, T., and Shen, C. (2020). “Cloud-based interactive database management suite integrated with deep learning-based annotation tool for landslide mapping.” in Proc. AGU Fall Meeting Abstr., vol. 2020, 2020, Art. no. NH030-0011.
- Nagendra, S., Manjunatha, B., Shen, C., Kifer, D., Pei, T. (2020). “An efficient deep learning mechanism for cross-region generalization of landslide events.” in Proc. of AGU Fall Meeting Abstr., vol. 2020, 2020, Art. no. NH030-0010.
Awards
- Department of Energy (DOE) Award # DE-SC0025738, "Resilience to Growing Extreme Natural Hazards: Developing a Northeast Hazards Workforce." Co-PI
- National Science Foundation (NSF) Award # RISE-2425802, "Unifying Landslide Domain Knowledge and AI to Understand Landslide Causality." PI
- 2024 CUNY Interdisciplinary Research Grant, "Empowering Landslide Prediction and Community Resilience with Physics-Guided Knowledge Transfer." PI
- PSC-CUNY Research Award Program, "Towards Reliable Data Science Applications in Landslide Prediction: Systematic Investigation into Data Dependencies to Create Widespread Community Awareness." PI